COMBINE – sustainable ConditiOn Monitoring of wind turBines using sound sIgnals and machiNe lEarning techniques
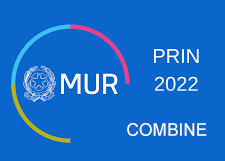
Durata: 28 Settembre 2023 – 27 Settembre 2025 (2 anni)
Ente finanziatore: Ministero dell’Università e della Ricerca (MUR) – PRIN 2022 – 20223LMSZN
Principal Investigator: Luca Fredianelli (IPCF)
Unità di ricerca coinvolte (referente): Università degli studi di Ferrara (Mattia Battarra); Università degli studi della Campania “Luigi Vanvitelli” (Gino Iannace); Università degli studi di Palermo (Francesco Guarino)
Settori ERC: PE8_6 Energy processes engineering; PE6_11 Machine learning, statistical data processing and applications using signal processing; PE6_7 Artificial intelligence, intelligent systems, natural language processing
Parole chiave:
Il PNRR dovrebbe incrementare la produzione italiana di energia eolica attraverso il repowering dei vecchi parchi eolici (WF). Sfortunatamente, questo processo viene eseguito sostituendo le vecchie turbine eoliche (WT) con turbine usate provenienti dal Nord Europa. Sebbene le sostituzioni migliorino la potenza, le WT rimangono prodotti vecchi e soggetti a un maggior rischio di guasti. Il progetto intende sviluppare uno strumento per l'identificazione dei guasti delle WT, anche in tempo reale, fornendo un allarme precoce. La metodologia si basa sul monitoraggio del suono emesso e sulla sua analisi con tecniche di Machine Learning (ML). Il risultato atteso del progetto è uno strumento semplice, economico e automatizzato che trova il suo impiego ideale nei WT di vecchia generazione, privi di sistemi di controllo per la diagnosi dei guasti a bordo. Lo strumento migliora la manutenzione riducendo i tempi di fermo dei WT e riducendo le ispezioni dirette sulla macchina, solitamente complesse e costose data la posizione remota dei WT. Per raggiungere gli obiettivi, il progetto inizia con la classificazione delle modalità di guasto del WT attraverso la stesura del documento Failure Mode and Effect Analysis (Analisi delle modalità e degli effetti dei guasti), seguito dalla definizione del sottoinsieme di guasti che dovrebbero influenzare il suono emesso. Questi risultati, insieme alla definizione dei protocolli di misura, rappresentano l'inizio di uno studio sperimentale esteso in cui saranno eseguite campagne multiple di misura del rumore e dei dati meteorologici presso diversi WF in Campania, Sicilia e Toscana, i cui proprietari hanno già dichiarato interesse a collaborare. Le prime misure saranno eseguite su WT che presentano danni noti e portano alla caratterizzazione dell'impronta acustica prodotta dai guasti. La procedura di riconoscimento automatico dei guasti saranno poi sviluppate attraverso algoritmi di ML applicati ai segnali acquisiti. Sarà sviluppato un tool di rimozione automatica degli eventi spuri dal suono misurato, che consente di alimentare la Rete Neurale Artificiale con i segnali acustici con il miglior rapporto segnale/rumore. La misurazione dell'immissione WT è notoriamente difficile e richiede tecniche specifiche. Infatti, l'immissione e i segnali residui possono sovrapporsi nel tempo e nella frequenza all'interno del rumore misurato e devono essere separati. In ogni punto di misura, il livello di immissioni sarà modellato in funzione della velocità di rotazione delle pale e il residuo in funzione della velocità del vento all'altezza del suolo, seguendo le linee guida ISPRA per il rumore dei WT, di cui il PI è coautore. Questo, combinato con i dati operativi e meteorologici forniti dagli operatori, consentirà di definire la WT sana. Per la fase di validazione verranno eseguite ulteriori campagne di misura. Le misure saranno ripetute nel corso dell'anno per includere la variazione stagionale del rumore della vegetazione indotto dal vento che, se non considerato, potrebbe produrre falsi positivi. La sostenibilità ambientale delle soluzioni proposte nel progetto sarà stata studiata anche attraverso la metodologia del Life Cycle Assessment secondo la norma ISO 14040.